2023 年十大人工智能(LLM)必读论文(下)
欢迎大家点击在看,或转发到朋友圈~~这样兔儿就更有动力发更多好内容了~ LLM
*本文 4400 字左右
作者:SEBASTIAN RASCHKA, PHD,原文名称:Ten Noteworthy AI Research Papers of 2023
2023 年,是机器学习和人工智能发展最为迅速的一年,这里和分享分享 10 篇笔者认为最值得关注的论文(今天是第二部分)。
上一篇见: 2023 年十大人工智能(LLM)必读论文(上)
当然,这里主要是以大语言模型(LLM)论文为主。选择标准可能也会稍显主观,但还是基于笔者个人特别喜欢或认为有影响力、值得关注的论文。( 注:这里的排序顺序是推荐阅读顺序,而不是根据质量或影响力排序)。
6)Mistral 7B 尽管 Mistral 7B 论文有些简短,但它提出的模式很有影响力:
简而言之,Mistral 7B 论文介绍了一个紧凑但功能强大的语言模型,尽管它的规模相对较小,只有 70 亿个 Token,但在各种 benchmark测试中,它的表现却优于更大的同类模型,比如它的表现超过了 13B Llama 2 模型。 ( 除了比其大两倍的 Qwen 14B 之外,Mistral 7B 也是今年 NeurIPS LLM Finetuning Efficiency 挑战赛获胜方案中使用的 base mode)

来自 https://arxiv.org/abs/2310.06825 的注释图,Mistral 7B 和 Llama 13B 的性能比较
从架构上来看,Mistral 7B 与 Llama 2 共享了 group-query attention,虽然与 Llama 2 非常相似,但 Mistral 架构中的一个有趣的补充是 sliding window attention ,用以节约内存并提高计算吞吐量,从而加快训练速度。 (Sliding window attention was previously proposed in Child et al. 2019 and Beltagy et al. 2020)

Zephyr 和 Mixtral


Mixture of Experts 101
如果你对 MOE 还比较陌生,可以参考下面的解释:
为什么选择 MoE?
结合起来,像 Mixtral 这样的 7B 模型中的 8 个Expert,总共有约 56B 个参数。实际上,它少于 56B,因为 MoE 方法仅应用于 FFN (feed forward network, aka fully-connected) ,而不是self-attention weight matrices。因此,它可能更接近 40-50B 个参数。请注意, Router 会 rerouteToken,这样在前向传播过程中一次只使用<14B 个参数(2x <7B,而不是全部<56B),因此与传统的非 MoE 方法相比,训练(尤其是 inference)将会更快。
如果想要了解更多关于 MOE 的内容,这里有一个 Sophia Yang 推荐的阅读清单:
- The Sparsely-Gated Mixture-of-Experts Layer (2017)
- GShard: Scaling Giant Models with Conditional Computation and Automatic Sharding (2020)
- MegaBlocks: Efficient Sparse Training with Mixture-of-Experts (2022)
- Mixture-of-Experts Meets Instruction Tuning (2023)
此外,如果读者对尝试 MoE 和 LLM 感兴趣,也可以查看 OpenMoE 的repository,在今年早些时候实现并分享了 MoE LLM 地址:https://github.com/XueFuzhao/OpenMoE

- Textbooks Are All You Need -- the phi-1 paper
- Textbooks Are All You Need II: phi-1.5 Technical Report
- The Phi-2: The Surprising Power of Small Language Models announcement
7) Orca 2: Teaching Small Language Models How to Reason
这是一篇相对较新的论文,因此时间会证明它是否会在未来数月或数年内对我们如何训练 LLM 产生持久的影响。
收录这篇论文的原因在于:它融合了多个概念和观点:
它是如何工作的?简而言之,主要分为 4 个步骤:
- 用一组人类编写的指令(本例中为 175 条)和示例指令建立任务池;(Seed task pool with a set of human-written instructions (175 in this case) and sample instructions;)
- 使用预训练 LLM(如 GPT-3)确定任务类别;( Use a pretrained LLM (like GPT-3) to determine the task category)
- 给定新指令,让经过预训练的 LLM 生成响应(Given the new instruction, let a pretrained LLM generate the response)
- 收集、修剪和过滤回复,然后将其添加到任务池中(Collect, prune, and filter the responses before adding them to the task pool)




在图像和视频中的Object recognition和segmentation,以及分类和生成建模,是计算机视觉的主要研究领域。
简要说明这两项任务之间的区别:Object recognition是预测边界框和相关标签;segmentation则是对每个像素进行分类,以区分前景和背景物体:


The Segment Anything Model 三个主要组成部分,见https://arxiv.org/abs/2304.02643 稍微详细一点,这三个部分可以总结如下:
- An image encoder utilizing a masked autoencoder based on a pretrained vision transformer (ViT) that can handle high-resolution inputs. This encoder is run once per image and can be applied before prompting the model
- A prompt encoder that handles two types of prompts: sparse (points, boxes, text) and dense (masks). Points and boxes are represented by positional encodings combined with learned embeddings for each prompt type. And free-form text uses an off-the-shelf text encoder from CLIP. Dense prompts, i.e., masks, are embedded using convolutions and summed element-wise with the image embedding.
- A mask decoder maps the image embedding, prompt embeddings, and an output token to a mask. This is a decoder-style transformer architecture that computes the mask foreground probability at each image location.
图像segmentation对于自动驾驶汽车、医学成像等应用至关重要。在短短 6 个月内,该论文已经被引用超过 1500 次,并且已经有许多项目在这篇论文的基础上构建。
10) Align your Latents: High-Resolution Video Synthesis with Latent Diffusion Models
Emu 视频:通过显式图像条件分解文本到视频生成,是 Meta 研究部门的另一个值得注意的计算机视觉项目。Emu 是一个可以从文本提示生成整个视频的文本到视频模型, 虽然它不是第一个令人印象深刻的文本到 视频生成模型,但它与以前的作品相比有很大优势:

新年快乐!
新年快乐!2024 大展宏图,越来越好!
【阅读更多】
6000 字解读:当前大语言模型 LLM 研究的 10 大挑战
2023 年十大人工智能(LLM)必读论文(上)
最新!彻底颠覆 Office,微软发布 Copil
生成式 AI 颠覆前端,你该何去何从?
重磅突发!OpenAI 正式推出多模态 GPT-4
免责声明:文章中的所有内容仅代表作者的观点,与本平台无关。用户不应以本文作为投资决策的参考。
你也可能喜欢
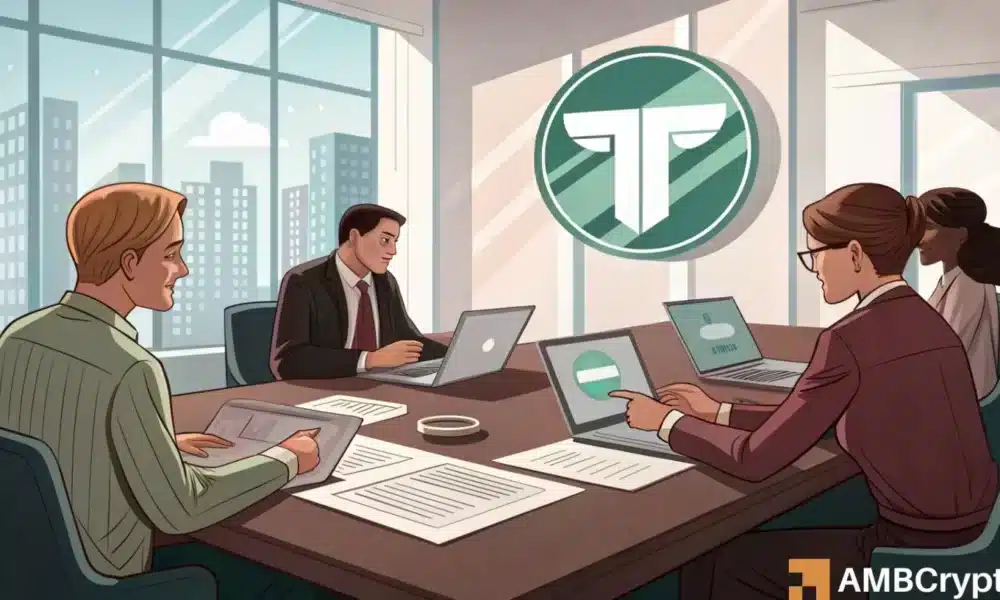
得州SB21法案升级:比特币储备扩容至5000亿市值代币,摩根大通警告‘监管套利风险’
近期,加密货币领域迎来重大消息,得州SB21法案升级让比特币储备扩容至5000亿市值代币,与此同时,摩根大通也发出了‘监管套利风险’的警告。这一事件引发了市场的广泛关注,下面我们将对这一热点事件进行全面解析。得州SB21法案解读得州
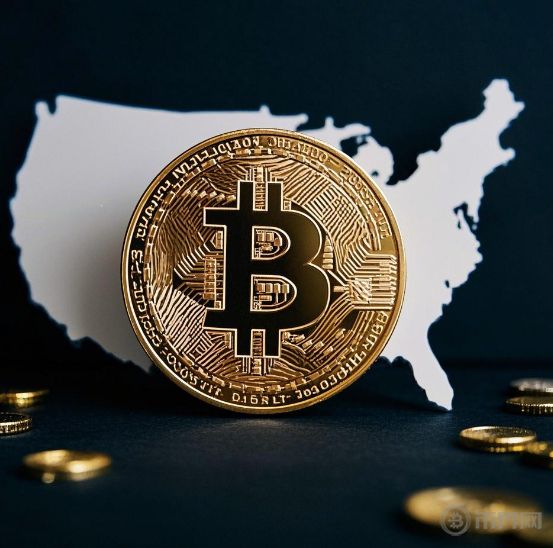
20州立法潮冲击美联储霸权?Satoshi Action曝特朗普团队密谋‘国家比特币战略储备’
在加密货币领域,近期一则消息引发了轩然大波:20州立法潮冲击美联储霸权?Satoshi Action曝特朗普团队密谋‘国家比特币战略储备’。这一系列事件背后到底隐藏着怎样的玄机,又会对全球金融市场产生何种影响呢?让我们一同深入探究。20州
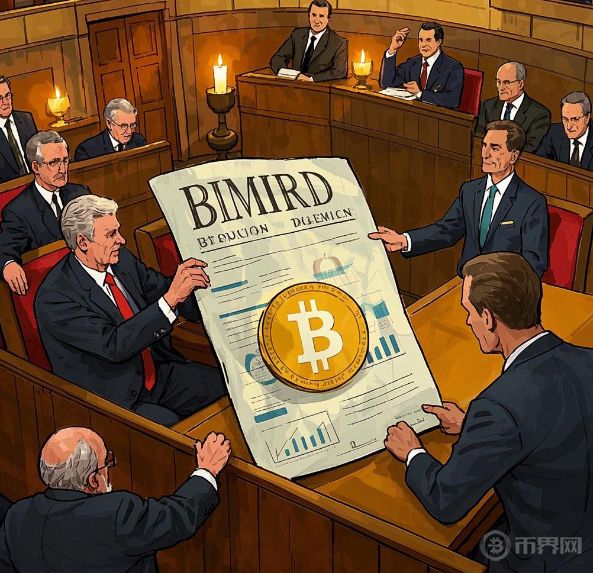
如果比特币市值达到$ 10T,这是Dogecoin的价格
如果比特币市值达到100万亿美元,该分析将探索Dogecoin的潜在价格上涨,到2030年预测,预测会显着增长。
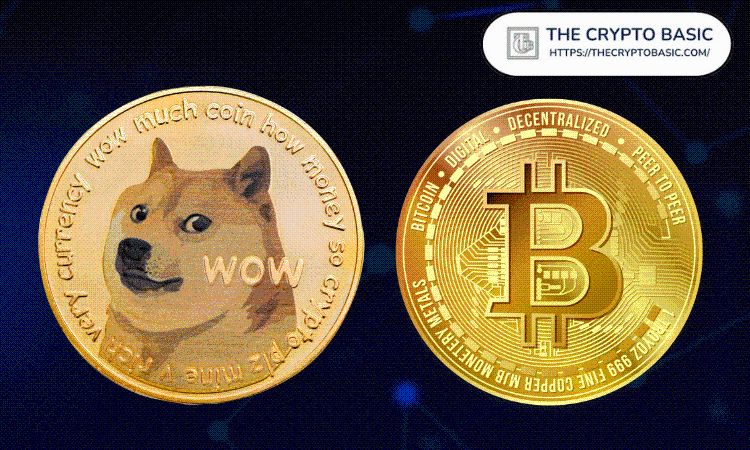
加密货币价格
更多








