Bitget:日次取引量の世界ランキングでトップ4にランクイン!
BTCマーケットシェア61.05%
Bitgetの新規上場:Pi Network
アルトコインシーズン指数:0(ビットコインシーズン)
BTC/USDT$92170.00 (-3.86%)恐怖・強欲指数25(恐怖)
ビットコイン現物ETFの純流入総額(-$50.4M(1日)、-$626.2M(7日))。6,200 USDT相当の新規ユーザー向けウェルカムギフトパッケージ。今すぐ獲得する
Bitgetアプリでいつでもどこでも取引しましょう今すぐダウンロードする
Bitget:日次取引量の世界ランキングでトップ4にランクイン!
BTCマーケットシェア61.05%
Bitgetの新規上場:Pi Network
アルトコインシーズン指数:0(ビットコインシーズン)
BTC/USDT$92170.00 (-3.86%)恐怖・強欲指数25(恐怖)
ビットコイン現物ETFの純流入総額(-$50.4M(1日)、-$626.2M(7日))。6,200 USDT相当の新規ユーザー向けウェルカムギフトパッケージ。今すぐ獲得する
Bitgetアプリでいつでもどこでも取引しましょう今すぐダウンロードする
Bitget:日次取引量の世界ランキングでトップ4にランクイン!
BTCマーケットシェア61.05%
Bitgetの新規上場:Pi Network
アルトコインシーズン指数:0(ビットコインシーズン)
BTC/USDT$92170.00 (-3.86%)恐怖・強欲指数25(恐怖)
ビットコイン現物ETFの純流入総額(-$50.4M(1日)、-$626.2M(7日))。6,200 USDT相当の新規ユーザー向けウェルカムギフトパッケージ。今すぐ獲得する
Bitgetアプリでいつでもどこでも取引しましょう今すぐダウンロードする

Bonk on ETHの価格BONK
決済通貨:
USD
$0.{9}6992-6.57%1D
価格チャート
最終更新:2025-02-25 01:48:19(UTC+0)
時価総額:--
完全希薄化の時価総額:--
24時間取引量:$377.69
24時間取引量 / 時価総額:0.00%
24時間高値:$0.{9}7484
24時間安値:$0.{9}6992
過去最高値:$0.{7}5896
過去最安値:$0.{9}6992
循環供給量:-- BONK
総供給量:
100,000,000,000,000BONK
流通率:0.00%
最大供給量:
--BONK
BTCでの価格:0.{4}1776 BTC
ETHでの価格:0.{12}2799 ETH
BTC時価総額での価格:
--
ETH時価総額での価格:
--
コントラクト:
0x4fba...edc9b64(Ethereum)
もっと
Bonk on ETHに投票しましょう!
注:この情報はあくまでも参考情報です。
今日のBonk on ETHの価格
Bonk on ETH の今日の現在価格は、(BONK / USD)あたり$0.{9}6992 で、現在の時価総額は$0.00 USDです。24時間の取引量は$377.69 USDです。BONKからUSDの価格はリアルタイムで更新されています。Bonk on ETH は-6.57%過去24時間で変動しました。循環供給は0 です。
BONKの最高価格はいくらですか?
BONKの過去最高値(ATH)は2024-05-11に記録された$0.{7}5896です。
BONKの最安価格はいくらですか?
BONKの過去最安値(ATL)は2025-02-24に記録され$0.{9}6992です。
Bonk on ETHの価格予測
2026年のBONKの価格はどうなる?
BONKの過去の価格パフォーマンス予測モデルによると、BONKの価格は2026年に$0.{9}9225に達すると予測されます。
2031年のBONKの価格はどうなる?
2031年には、BONKの価格は+26.00%変動する見込みです。 2031年末には、BONKの価格は$0.{8}2165に達し、累積ROIは+209.63%になると予測されます。
Bonk on ETHの価格履歴(USD)
Bonk on ETHの価格は、この1年で-95.08%を記録しました。直近1年間のUSD建ての最高値は$0.{7}5896で、直近1年間のUSD建ての最安値は$0.{9}6992でした。
時間価格変動率(%)
最低価格
最高価格 
24h-6.57%$0.{9}6992$0.{9}7484
7d-7.36%$0.{9}6992$0.{9}7503
30d-59.65%$0.{9}6992$0.{8}1702
90d-79.02%$0.{9}6992$0.{8}3666
1y-95.08%$0.{9}6992$0.{7}5896
すべての期間-95.08%$0.{9}6992(2025-02-24, 今日 )$0.{7}5896(2024-05-11, 290 日前 )
Bonk on ETHの市場情報
Bonk on ETHの集中度別保有量
大口
投資家
リテール
Bonk on ETHの保有時間別アドレス
長期保有者
クルーザー
トレーダー
coinInfo.name(12)のリアル価格チャート
Bonk on ETHの評価
コミュニティからの平均評価
4.4
このコンテンツは情報提供のみを目的としたものです。
BONKから現地通貨
1 BONK から MXN$01 BONK から GTQQ01 BONK から CLP$01 BONK から UGXSh01 BONK から HNLL01 BONK から ZARR01 BONK から TNDد.ت01 BONK から IQDع.د01 BONK から TWDNT$01 BONK から RSDдин.01 BONK から DOP$01 BONK から MYRRM01 BONK から GEL₾01 BONK から UYU$01 BONK から MADد.م.01 BONK から OMRر.ع.01 BONK から AZN₼01 BONK から SEKkr01 BONK から KESSh01 BONK から UAH₴0
- 1
- 2
- 3
- 4
- 5
最終更新:2025-02-25 01:48:19(UTC+0)
Bonk on ETH(BONK)の購入方法
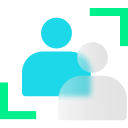
無料でBitgetアカウントを作成します
Eメールアドレス/携帯電話番号でBitgetに登録し、アカウントを保護するために強力なパスワードを作成します。
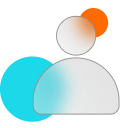
アカウントを認証する
個人情報を入力し、有効な写真付き身分証明書をアップロードして本人確認(KYC認証)を行います。
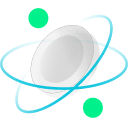
Bonk on ETH (BONK)を購入
BitgetでBonk on ETHを購入するには、様々なお支払い方法をご利用いただけます。
エリートトレーダーをフォローして、BONKのコピートレードを始めましょう。
Bitgetに登録し、USDTまたはBONKトークンを購入した後、エリートトレーダーをフォローしてコピートレードを開始することもできます。
Bonk on ETHのニュース
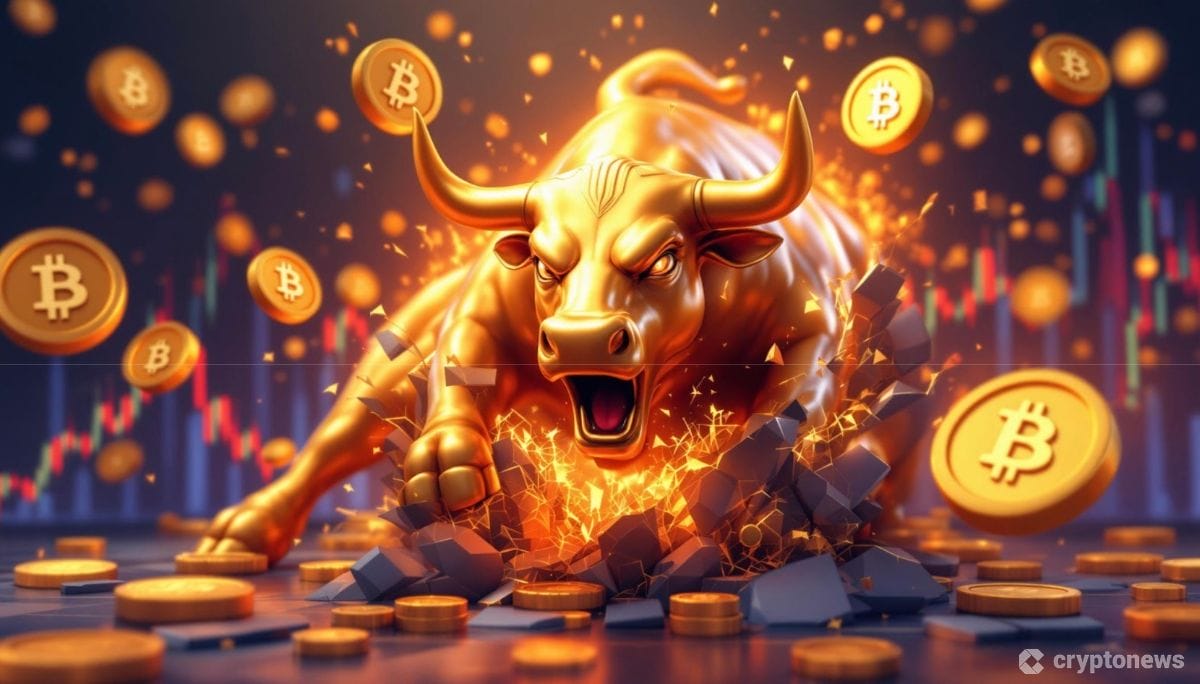
2025年のミームコイン市場 、専門家が注目する新興銘柄とは
Cryptonewsは、10年以上にわたる暗号資産(仮想通貨)の報道経験に裏付けされた、信頼に足る洞察を提供しています。経験豊富なジャーナリストやアナリストが、深い知識を駆使し、ブロックチェーン技術を実際に検証しています。厳格な編集ガイドラインを遵守し、仮想通貨プロジェクトについて、正確かつ公正な報道を徹底しています。長年の実績と質の高いジャーナリズムへの取り組みにより、Cryptonewsは暗号資産市場の信頼できる情報源となっています。会社概要も併せてご覧ください。 広告開示私たちは、読者の皆様に対し、完全な透明性を提供することを重要視しています。当サイトの一部のコンテンツにはアフィリエイトリンクが含まれており、これらのリンクを通じて発生した取引に基づき、当社が手数料を受け取る場合がございます。
CryptoNews•2025-02-20 00:55
Glassnode: ミームセクターにおけるオープン契約の規模が大幅に縮小し、DOGEの7日間平均の下落率は58.45%に達した
Bitget•2025-02-19 12:30
CMEグループ、2024年Q4に仮想通貨取引が過去最高を記録
cointelegraph-jp-crypto•2025-02-13 07:22
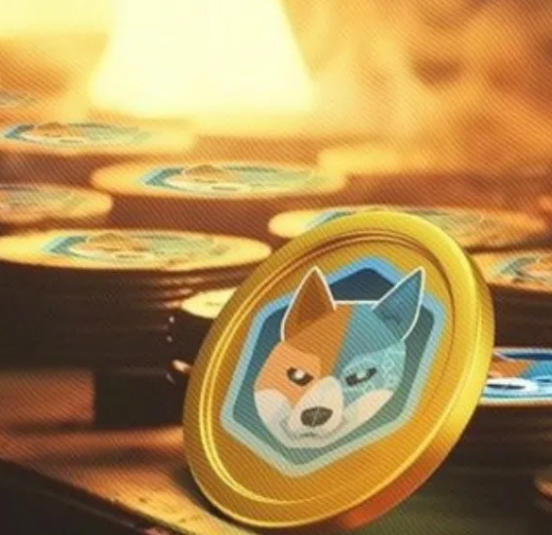
VC に対する考え、Meme に対する考え、暗号通貨の世界の次の波は左か右か?
cointime-jp-news•2025-02-12 06:48
40%下落後に反転アラートを示すBONK、 2兆トークンのバーン完了
Yellow•2025-02-09 23:44
もっと購入する
よくあるご質問
Bonk on ETHの現在の価格はいくらですか?
Bonk on ETHのライブ価格は$0(BONK/USD)で、現在の時価総額は$0 USDです。Bonk on ETHの価値は、暗号資産市場の24時間365日休みない動きにより、頻繁に変動します。Bonk on ETHのリアルタイムでの現在価格とその履歴データは、Bitgetで閲覧可能です。
Bonk on ETHの24時間取引量は?
過去24時間で、Bonk on ETHの取引量は$377.69です。
Bonk on ETHの過去最高値はいくらですか?
Bonk on ETH の過去最高値は$0.{7}5896です。この過去最高値は、Bonk on ETHがローンチされて以来の最高値です。
BitgetでBonk on ETHを購入できますか?
はい、Bonk on ETHは現在、Bitgetの取引所で利用できます。より詳細な手順については、お役立ちの購入方法 ガイドをご覧ください。
Bonk on ETHに投資して安定した収入を得ることはできますか?
もちろん、Bitgetは戦略的取引プラットフォームを提供し、インテリジェントな取引Botで取引を自動化し、利益を得ることができます。
Bonk on ETHを最も安く購入できるのはどこですか?
戦略的取引プラットフォームがBitget取引所でご利用いただけるようになりました。Bitgetは、トレーダーが確実に利益を得られるよう、業界トップクラスの取引手数料と流動性を提供しています。
Bonk on ETH(BONK)はどこで買えますか?
動画セクション - 素早く認証を終えて、素早く取引へ
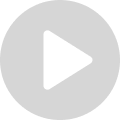
Bitgetで本人確認(KYC認証)を完了し、詐欺から身を守る方法
1. Bitgetアカウントにログインします。
2. Bitgetにまだアカウントをお持ちでない方は、アカウント作成方法のチュートリアルをご覧ください。
3. プロフィールアイコンにカーソルを合わせ、「未認証」をクリックし、「認証する」をクリックしてください。
4. 発行国または地域と身分証の種類を選択し、指示に従ってください。
5. 「モバイル認証」または「PC」をご希望に応じて選択してください。
6. 個人情報を入力し、身分証明書のコピーを提出し、自撮りで撮影してください。
7. 申請書を提出すれば、本人確認(KYC認証)は完了です。
Bitgetを介してオンラインでBonk on ETHを購入することを含む暗号資産投資は、市場リスクを伴います。Bitgetでは、簡単で便利な購入方法を提供しており、取引所で提供している各暗号資産について、ユーザーに十分な情報を提供するよう努力しています。ただし、Bonk on ETHの購入によって生じる結果については、当社は責任を負いかねます。このページおよび含まれる情報は、特定の暗号資産を推奨するものではありません。
Bitgetインサイト
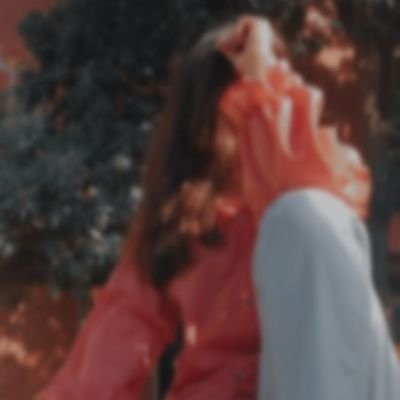
Samina_Khan
2日
THREE ALTCOINS SHOWING STRONG MARKET POTENTIAL
The crypto market continues to present opportunities for high returns, with Solana (SOL), Sui (SUI), and Bonk (BONK) currently displaying notable market activity. Here’s an overview of their latest movements and potential trends.
SOLANA (SOL): NETWORK UPGRADES AND PARTNERSHIPS
Current Price: $236.40
Market Cap: $112.24 billion
Solana remains one of the leading blockchain networks, with ongoing technological upgrades and partnerships supporting its growth. Market interest continues to drive activity, and current trends suggest potential for increased price movements.
SUI (SUI): HIGH TRADING VOLUME AND ADOPTION
Current Price: $3.54
Market Cap: $10.07 billion
24-Hour Trading Volume: $1.52 billion
SUI has seen a high volume-to-market cap ratio of 14.99%, indicating strong market participation. The rising adoption rate and liquidity suggest continued investor interest, with potential for notable price action.
BONK (BONK): COMMUNITY-DRIVEN GROWTH
Current Price: $0.00005205
Market Cap: $3.9 billion
BONK has gained traction as a community-driven asset, with significant trading volume and whale activity. The current market trends highlight ongoing engagement and potential volatility.
MARKET OUTLOOK
These altcoins have shown high liquidity, active trading, and growing adoption. While price movements depend on various market factors, their recent trends indicate continued interest from traders and investors.
SOL-0.95%
BONK-0.76%
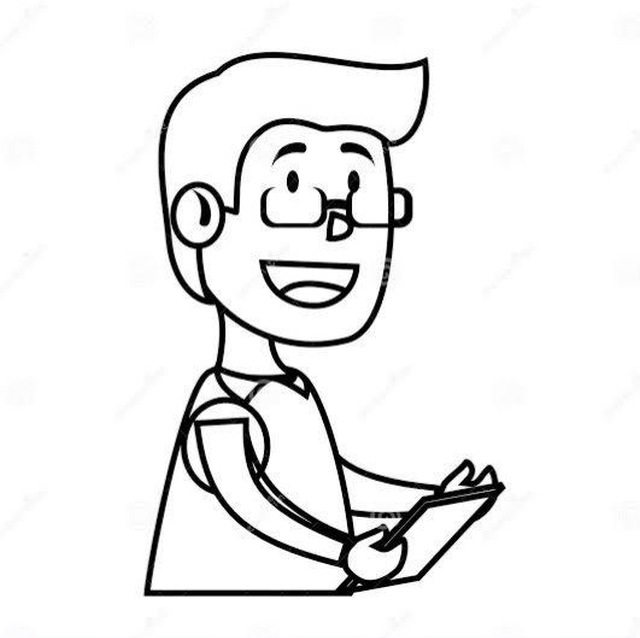
Miles_Bitget
2025/02/20 00:51
$BONK price reacted to the 1.38 Fib extension level to the downside.
For now, still no evidence that the price has formed a low in wave-(iv). A break above $0.0000207 would be the first sign that wave-3 is unfolding to the upside. Resistance is between $0.000021 and $0.000026.
BONK-0.76%
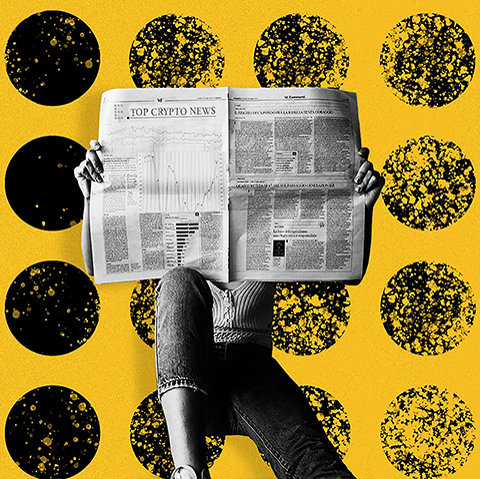
TopCryptoNews
2025/02/19 17:45
🐸 $PEPE Faces Major Deleveraging Amid 42% Drop
According to on-chain analytics platform Glassnode, frog-themed cryptocurrency PEPE has seen a major deleveraging as Futures Open Interest across the meme coin sector has dropped significantly since their peaks.
Recently, a trend has emerged among meme coins, with open interest cooling off following speculative peaks. The trend is clear: open interest has sharply corrected across meme coins. This can point to leverage flushing out but also signal a shift in sentiment across the meme coin sector.
💬 PEPE, BONK, and WIF also saw major deleveraging:
📉 PEPE: -71.93% ($1.25B → $351M)
📉 BONK: -75.10% ($715M → $178M)
📉 WIF: -69.83% ($653M → $197M)
These memecoins followed similar patterns, with OI cooling off after speculative peaks.— glassnode February 19, 2025
Frog-themed meme coin PEPE has seen its price drop by 42% monthly and experienced a major deleveraging, with its futures open interest plunging by 71.93% — falling from $1.25 billion to $351 million.
The meme coin sector, in general, is undergoing a significant shift, with futures open interest (OI) sharply contracting after reaching speculative peaks. This trend is reflected in other meme coins such as SHIB, DOGE, WIF and BONK.
At the height of the speculative frenzy, meme coins saw skyrocketing trade volumes and massive leveraged holdings. However, recent data shows that this exuberance is cooling off. The substantial drop in futures open interest across the sector indicates that traders are stepping back, reducing their exposure and flushing out excessive leverage from the market.
PEPE down 42% monthly
After a stellar surge in late 2024, PEPE is now facing a harsh reality. The token has experienced a steep 42% drop over the past month, mirroring a broader correction in the often volatile meme coin sector.
At press time, PEPE was down 2.29% in the last 24 hours to $0.0000092 and down 0.09% weekly.
PEPE has steadily declined since reaching an all-time high of $0.000028 on Dec. 9, 2024, and is now down 66.5% from this peak. PEPE declined all through January, dropping for four consecutive weeks at a stretch.
Bears took a breather in the past week, with PEPE ending the week in the green, but the drop has now resumed, with PEPE down this week. Eyes will be on recovery for the PEPE price beyond the daily SMA 50 at $0.00001453 to ascertain a price comeback.
#PEPE #Pepecoin
DOGE-0.31%
WIF-2.15%
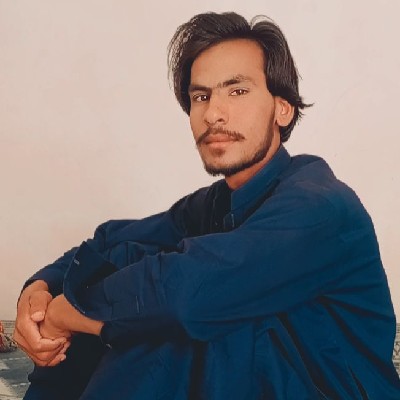
Shoiabaktar37
2025/02/19 08:54
Hier ist eine Zusammenfassung der Ethereum-basierten Meme-Coin-Projekte:
Meme-Coin-Projekte
1. *Shiba Inu (SHIB)*: Ein etabliertes Projekt mit einer starken Community und Ökosystem.
2. *Bonk (BONK)*: Ein community-getriebenes Projekt mit Integration in DeFi-Projekte und mehreren Blockchain-Netzwerken.
3. *Pepe (PEPE)*: Ein Projekt mit starker Community-Unterstützung und viralen Appeal.
SHIB+0.95%
PEPE-0.76%
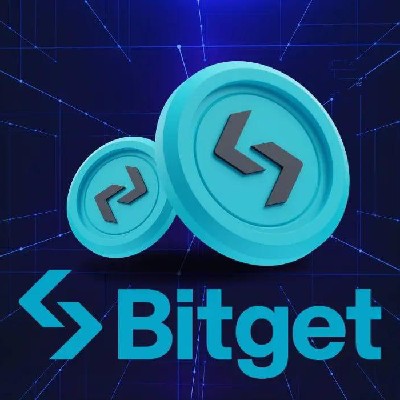
FaithyMaria-BTC
2025/02/19 08:15
Ethereum Meme Coins Poised for Potential 100x Gains Before February Ends
As the cryptocurrency market continues to evolve, Ethereum-based meme coins have emerged as a dynamic and high-reward segment. These tokens, often inspired by internet culture and viral trends, have captivated investors with their potential for exponential growth. With February nearing its end, several Ethereum meme coins are generating buzz for their potential to deliver significant returns. Below, we explore some of these promising tokens.
1. Shiba Inu (SHIB)
Launched in August 2020, Shiba Inu (SHIB) has established itself as a prominent player in the meme coin arena. Dubbed the "Dogecoin killer," SHIB has cultivated a robust community and ecosystem. Over the past year, SHIB's value has surged significantly, reflecting growing interest and adoption. It continues to maintain momentum, making it a strong candidate for potential gains.
2. Bonk (BONK)
Bonk (BONK) is a meme coin that has garnered attention due to its unique community-driven approach. Despite recent price fluctuations, BONK has maintained a dedicated following. Its integration into various decentralized finance (DeFi) projects and its presence on multiple blockchain networks position it as a contender for significant growth.
3. Pepe (PEPE)
Inspired by the popular internet meme "Pepe the Frog," Pepe (PEPE) aims to carve out its niche in the meme coin sector. It has seen remarkable growth over the past year, fueled by strong community engagement and viral appeal. While price movements can be volatile, its popularity keeps it in the spotlight for potential gains.
4. Floki Inu (FLOKI)
Floki Inu (FLOKI) has captured the imagination of crypto enthusiasts with its unique blend of humor, community engagement, and bold innovation. Named after the adventurous Viking, FLOKI embodies a spirit of exploration and determination, resonating with investors who appreciate both a playful approach and serious growth potential in the crypto market. Its vibrant community and ongoing developments make it a meme coin with promising prospects.
5. Baby Doge Coin (BABYDOGE)
Building on Dogecoin's legacy, Baby Doge Coin (BABYDOGE) offers improved transaction speeds and integrates charitable initiatives to give back to communities. With a passionate fanbase and a commitment to philanthropy, Baby Doge Coin has cemented itself as a feel-good investment that still packs serious potential for long-term gains.
🔻Conclusion
While the allure of substantial returns is enticing, it's crucial to approach investments in meme coins with caution. The market is highly volatile, and thorough research is essential. Engaging with the communities, understanding the tokenomics, and staying updated with project developments can provide valuable insights. As February draws to a close, these Ethereum-based meme coins present intriguing opportunities for those willing to navigate the risks inherent in the crypto landscape.
$SHIB $BONK $PEPE $FLOKI $BABYDOGE
DOGE-0.31%
FLOKI-1.21%
関連資産
同等の時価総額
すべてのBitget資産の中で、時価総額がBonk on ETHに最も近いのはこれらの8資産です。
