AI in Data Centers – Challenges and Solutions
HodlX Guest Post Submit Your Post
The exponential surge in demand for AI-powered applications in recent years has necessitated a new approach to data center design, configuration and management.
Wall Street Journal estimates around 20% of the global data center capacity is currently used for AI purposes.
However, with over 77% of companies already using or exploring AI technology, traditional data centers may be going obsolete
fast.The AI stand-off
Due to their complex algorithms and models, AI applications typically require more power and computing resources than others.
For example, a simple query on ChatGPT requires almost 10 times as much electricity needed to process a quick search on Google.
Traditional data centers are designed with an average density of five to 10 kilowatts per rack, but this increases to 60 or more kilowatts per rack to handle AI applications.
More workload and energy demands equals higher overhead costs.
In addition, data centers have to come up with alternative and advanced ways of dealing with cooling problems, vulnerabilities, security challenges and maintenance issues that can arise due to staffing shortages.
Then there is the question of environmental sustainability. Researchers estimate that GPT-3 generated over 552 tons of CO2 before it was even released for public use in 2020.
This figure is equivalent to the CO2 that would be produced by 123 gasoline vehicles over a full calendar year.
Unfortunately, unless these challenges are strategically and dynamically addressed, we may be looking at an infrastructural tight-rope similar to the GPU supply deficit.
The shortage of data centers fully equipped to handle the overwhelming demands of AI technology may ultimately slow down growth, promote monopolization of AI infrastructure and have serious implications for the environment.
Building for now and the future
To tackle these problems headlong, many companies are already implementing new measures.
These include using collocated data centers to reduce operational costs, promote scalability and ensure the availability of skilled on-site maintenance.
Data centers are also employing more advanced cooling techniques like liquid cooling, direct-to-chip cooling and immersive cooling, as opposed to conventional air cooling systems.
For new centers, design becomes paramount. For example, in 2022, Meta paused the construction of its $800 million data center in Texas to consider redesigning the 900,000-square-foot facility.
However, beyond just functioning as the infrastructural and computing powerhouse for AI-backed applications and products, data centers can also leverage the same AI to optimize performance, manage costs and ensure operational efficiency in several ways.
Let’s take a look at some of them.
Workload management
AI and automation tools can precisely predict and allocate workloads more efficiently in data centers, ensuring that deployments match resource requirements.
This reduces waste by minimizing the under-utilization of computing hardware and reducing energy consumption. Over 32% of cloud spending is wasted mostly due to over-provisioning.
AI systems, however, can redistribute resources to projects that need them the most, optimizing performance and maximizing idle hardware.
Repetitive and routine tasks can be conveniently automated, saving time, energy and skilled manpower.
AI can also process data and performance metrics, allowing for strategic, proactive measures to address potential workload management problems before they occur.
AI-driven cooling systems
In addition to introducing better cooling facilities, AI can play a significant role in dynamically detecting and adjusting temperature.
Instead of statically cooling hardware in the data center, AI can analyze and act on temperature data to supply just the needed amount of cooling to each hardware.
This can regulate humidity conditions for optimal performance, improve power efficiency and prolong the use-life of equipment.
Dynamic power usage effectiveness
Real-time monitoring and predictive analytics by AI systems can provide key insights into power usage patterns as well as inefficiencies, allowing managers to make data-backed decisions and implement necessary power management strategies.
While the objective fact remains that power requirements for data centers running AI workloads will always be invariably higher than traditional data centers, the synergistic efforts of AI-driven management and data center design can make a significant impact.
Data centers can also minimize their carbon footprint and reduce environmental impact by prioritizing efficient energy management systems and adopting power management techniques like DVFS (dynamic voltage and frequency scaling).
Rounding up
The price for a highly sophisticated digital future lies in the core of infrastructure.
Data centers must adopt physical, operational and software changes to keep up with the evolving modern world and its AI demands.
Thankfully, AI challenges can also be addressed with AI solutions.
As the tech industry gradually adapts and technology improves, AI-driven workload management and optimization will become mainstream, leading to robust data centers equipped to power the future.
Innovation from other alternatives like decentralized computing infrastructure will also create healthy competition and improve efficiency.
Daniel Keller is the CEO of InFlux Technologies . He has more than 25 years of IT experience in technology, healthcare and nonprofit/charity works. Daniel successfully manages infrastructure, bridges operational gaps and effectively deploys technological projects.
Generated Image: Midjourney
coin_news.disclaimer
coin_news.may_like
Chan Ho-lim: Hong Kong has strong potential for Web3 development
US spot Bitcoin ETF had a net outflow of $54.93 million yesterday
Nvidia to be included in Dow Jones
coin_news.trending_news
coin_news.morecoin_news.crypto_prices
coin_news.more






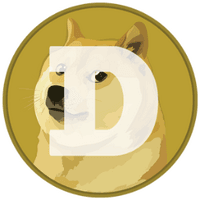

